Research topics
Robot learning
Robot learning is a multidisciplinary field that intersects robotics, machine learning, and artificial intelligence, and is key to the learning of complex behaviors on high degree of freedom systems like humanoid robots. Based on our extensive work on imitation learning, statistical generalization of sensorimotor experience and trajectory libraries, and reinforcement learning, we continue our work in this domain. Our efforts combine all major fields of robotic learning, such as imitation learning, reinforcement learning, supervised, unsupervised and self-supervised learning, deep learning, transfer learning including sim2real, etc.
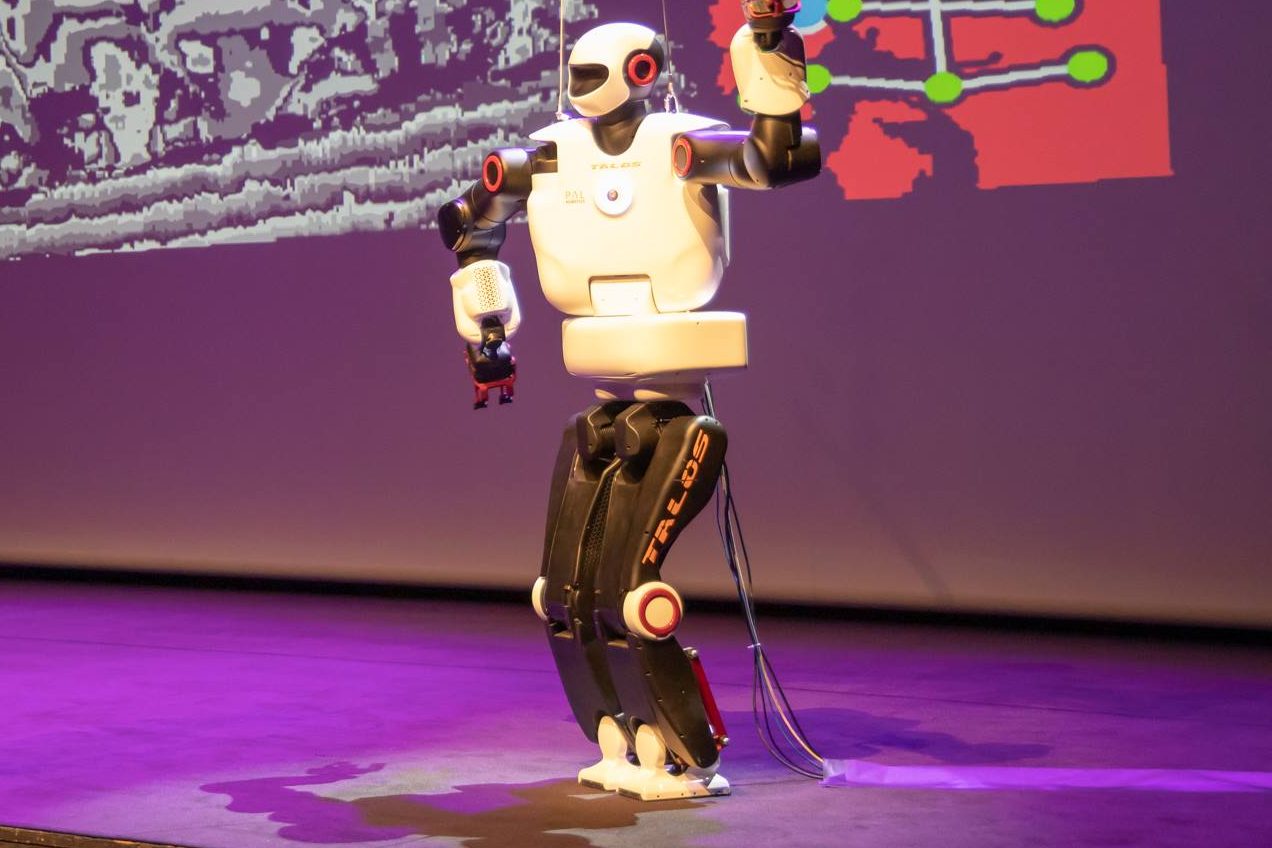
Robot perception and actions
In the area of robot perception we study approaches that combine active vision and manipulation with the processing of visual information and other sensing modalities including force. By active sensing we can bring in additional information that can be exploited to autonomously learn new sensory representations and object affordances, which would be very difficult or even impossible with static perceptual systems. Lately, we combine this with large language models and vision language (foundation) models for robotics, as the latest AI approaches in the field. We are also interested in the development of human-like visual systems to improve the sensing capabilities of our robots.
Advanced applications
We apply our knowledge to implement advanced solutions beyond the current state of robot applications both in terms of the complexity of actions and approaches to solving difficult problems with robots in everyday and industrial settings. An important line of our work is reconfigrable robotics – we introduce self-reconfigurable hardware and software solutions based on recofigurable robotics cells. The challenge of this is to provide methodologies for re-design of the robot cell including the location of robots and other elements in the workcell and the choice of grippers and sensing systems.
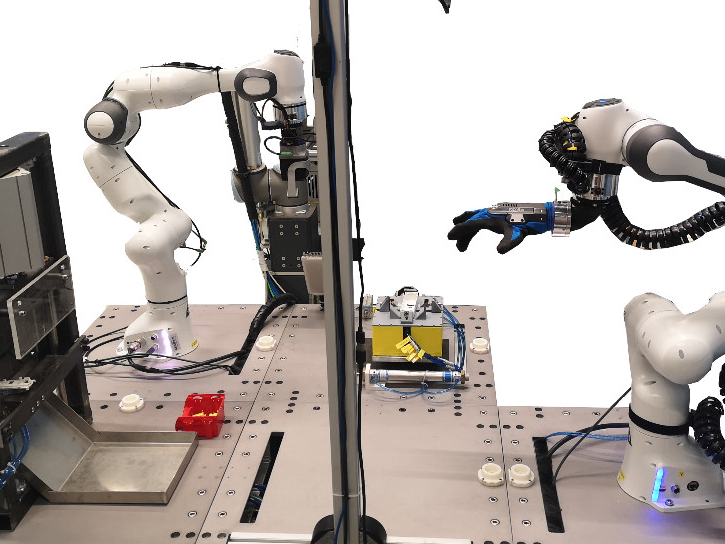
On the software side, approaches for fast re-programming and adaptation of manipulation actions for soft robots and grippers suitable for the given tasks need to be provided. Soft components make grasping and compliant control easier, but they can be problematic in tasks that usually rely on high precision position control.
Handling of soft, deformable materials, goes beyond typical complexity in robotic applications and advances in robotic manipulation of deformable objects have lagged behind work on rigid objects due to the far more complex dynamics and configuration space. We advance the state of the art of perception/inspection and robotic manipulation of textile and fabric, in order to bridge the technological gap and enable automation of such material handling.
Other topics
Besides in these key areas, the members of HCR conduct research in a number of related fields including kinematics, motor control, execution of collaborative behaviors (both human-robot and robot-robot), where there exists physical contact between agents, modeling of biological systems, visual tracking, object recognition and other areas of robotics, cognitive robotics and AI.